Harnessing the Power of Data Labeling in Machine Learning
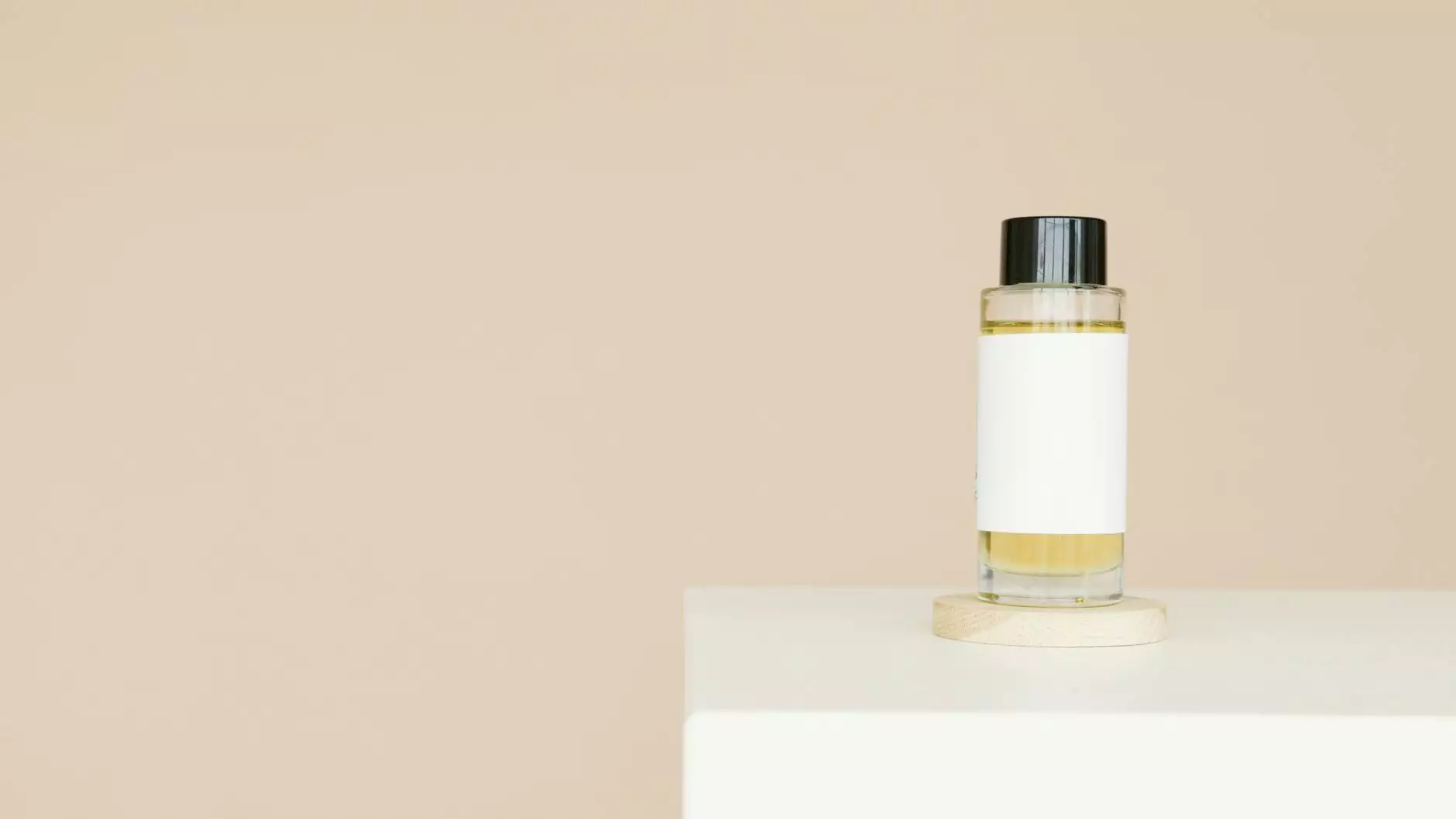
In today's data-driven world, the significance of data labeling in machine learning cannot be overstated. As companies and organizations increasingly rely on machine learning models to drive important decisions, the demand for accurately labeled data is at an all-time high. This article delves deep into the realm of data annotation, exploring its relevance, methodologies, and how platforms like KeyLabs.ai are revolutionizing the process.
Understanding Data Labeling in Machine Learning
Data labeling is the process of identifying and marking data points in a dataset to create a ground truth for machine learning models. It serves as the foundational step for any successful machine learning project. Without properly labeled data, models may struggle to learn patterns and, thus, fail to deliver actionable insights.
The Importance of Data Labeling
Quality data labeling is critical for several reasons:
- Model Accuracy: Accurate labels allow the model to learn from relevant features, ultimately improving prediction accuracy.
- Generalization: Well-labeled data enables models to generalize better to unseen data, reducing overfitting.
- Efficiency: Labeled data accelerates the training process, enabling quicker deployment of AI solutions.
- Insights and Decision Making: Properly labeled datasets provide better insights, helping in making informed business decisions.
Challenges in Data Annotation
While data labeling is pivotal, it comes with its own set of challenges:
- Scalability: As datasets grow, labeling them becomes increasingly time-consuming and labor-intensive.
- Consistency: Different annotators may interpret the same data points differently, leading to inconsistencies in labeling.
- Quality Assurance: Ensuring the accuracy and quality of labeled data requires a robust system for review and validation.
- Domain Expertise: Some projects require domain-specific knowledge for accurate labeling, making it challenging to find qualified annotators.
Key Data Annotation Techniques
There are several methods for data labeling in machine learning, including:
- Manual Annotation: Human annotators label the data, providing high accuracy but at a slower pace.
- Automated Annotation: Tools and algorithms pre-label data based on learned patterns, which can be reviewed and corrected by humans.
- Crowdsourcing: Distributing labeling tasks among a large group of people to achieve faster results.
- Active Learning: An iterative approach where the model identifies uncertain data points that need labeling.
Data Annotation Tools and Platforms
The choice of the right data annotation platform can significantly enhance the efficiency and quality of your labeling process. KeyLabs.ai offers robust solutions designed to address the challenges of data labeling in machine learning.
KeyLabs.ai Data Annotation Tools
KeyLabs.ai provides a comprehensive suite of data annotation tools tailored to various industries. These tools include:
- Image and Video Annotation: Tools for labeling objects in images and videos, essential for computer vision applications.
- Text Annotation: Solutions for labeling text data, including sentiment analysis, entity recognition, and more.
- Audio Annotation: Tools designed for transcribing and labeling audio data, crucial for voice recognition technologies.
- Custom Annotation Solutions: Flexible tools that can be adapted to meet specific project needs.
Benefits of Using KeyLabs.ai for Data Annotation
Utilizing a specialized platform like KeyLabs.ai offers numerous advantages, including:
- High-Quality Labeling: Advanced algorithms ensure consistency and accuracy in data annotation.
- Scalability: Easily handle large volumes of data without compromising quality.
- Collaboration Tools: Features that facilitate teamwork among annotators and project managers.
- Fast Turnaround: Accelerates the labeling process, enabling quicker model training and deployment.
Real-World Applications of Data Labeling
Data labeling plays a pivotal role in various industries. Here are some real-world applications:
- Healthcare: Labeled medical images assist in training diagnostic models to identify diseases.
- Finance: Annotation of financial documents helps in automated fraud detection and risk assessment.
- Retail: Labeled customer interactions can enhance recommendation systems and improve customer service.
- Automotive: Labeled data for self-driving cars enables better object detection and recognition.
Future Trends in Data Annotation
The landscape of data labeling in machine learning is continually evolving. Here are some emerging trends:
- Increased Use of AI in Annotation: Leveraging AI to assist in the annotation process, reducing the workload on human annotators.
- More Focus on Data Privacy: Ensuring compliance with data protection regulations while collecting and annotating data.
- Improved Annotation Quality through Collaboration: Enhanced workflows allowing stakeholders to collaborate and review annotations in real-time.
- Shift Towards Synthetic Data: Using computer-generated data to train models, reducing reliance on real-world labeled datasets.
Conclusion
In conclusion, data labeling in machine learning is an essential process that significantly impacts the success of AI initiatives. As organizations strive for better insights and smarter decision-making, the demand for quality labeled data will continue to grow. Platforms like KeyLabs.ai are at the forefront of this transformation, providing cutting-edge tools and solutions to meet the challenges of data annotation.
For businesses looking to harness the power of machine learning, investing in efficient and accurate data labeling is paramount. With the right tools and methodologies, you can ensure that your machine learning projects are built on a solid foundation of quality data.
data labeling machine learning