Unveiling the Power of Annotation Tools for Images
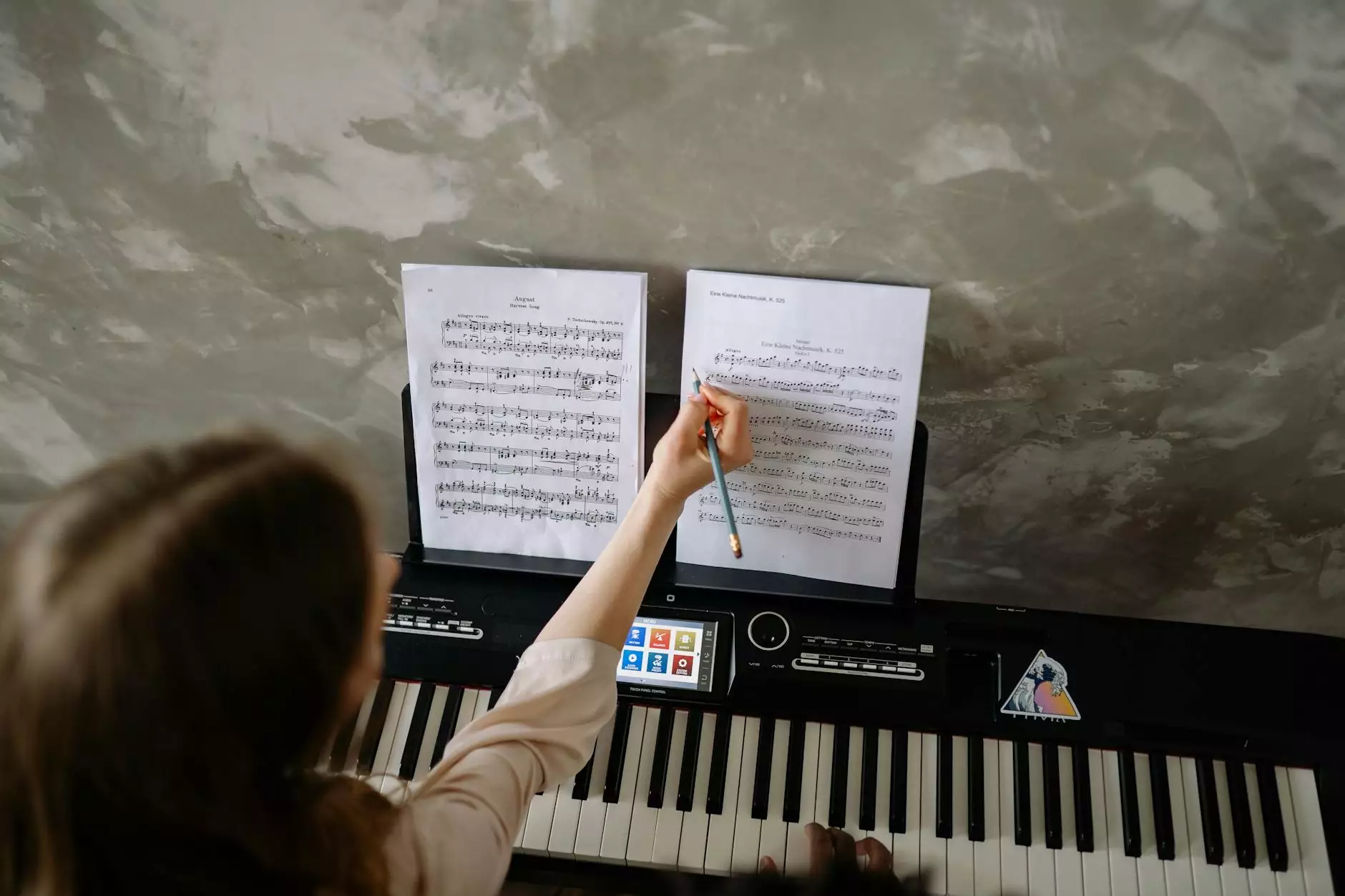
In the age of data, annotation tools for images play a pivotal role in the development of artificial intelligence and machine learning algorithms. As organizations increasingly rely on visual data to drive insights and decision-making, mastering the art of image annotation has become essential. This article explores what image annotation tools are, their benefits, and how they can transform raw data into valuable assets.
What Are Annotation Tools for Images?
Annotation tools for images provide users the capability to label and tag images with relevant information. This practice is not merely about drawing bounding boxes or placing tags; it encompasses a detailed process of enhancing the data’s usability for training AI models. Effective annotation can significantly improve the accuracy of machine learning predictions and insights derived from visual data.
Why Are Annotation Tools Essential?
The demand for annotation tools for images has surged as industries recognize their importance. Let's outline the key reasons:
- Enhanced Data Quality: Quality annotations lead to better training datasets, resulting in more accurate AI models.
- Efficient Workflow: Automated features in annotation tools can significantly reduce manual workload, allowing teams to work smarter.
- Scalability: These tools enable organizations to scale their data annotation processes to meet rising demands.
- Versatility: Annotation tools can be tailored for various types of image data, whether it's for medical imaging, autonomous vehicles, or retail.
- Collaboration: Modern annotation platforms facilitate teamwork, enabling multiple contributors to work on the same project seamlessly.
Types of Image Annotation Techniques
There are multiple methods to annotate images, each suited for different applications. Here are some widely used techniques:
1. Bounding Box Annotation
Bounding box annotation involves drawing rectangles around objects in an image. This method is commonly used in object detection tasks, where it’s crucial to identify and locate specific items.
2. Polygon Annotation
This technique allows users to create more complex shapes than bounding boxes. Polygon annotation is especially useful for applications requiring more detailed outlines, like segmentation tasks in medical imaging.
3. Semantic Segmentation
Semantic segmentation assigns a label to every pixel in an image, providing pixel-level classification. This method is essential for applications such as autonomous driving, where understanding the scene context is critical.
4. Keypoint Annotation
Keypoint annotation focuses on marking specific points of interest within an image, often used in gesture recognition and facial recognition tasks.
Key Features of Effective Annotation Tools
When evaluating annotation tools for images, several key features should be considered:
- User-Friendly Interface: The tool should be easy to navigate, allowing users to annotate images efficiently.
- Support for Multiple Formats: Compatibility with various image file formats enhances usability across different projects.
- Collaboration Features: Real-time collaboration capabilities can improve team productivity and communication.
- Quality Assurance Options: Built-in quality checks ensure that the annotations are accurate and consistent.
- Integration Support: The ability to integrate with other software tools and platforms streamlines workflows.
Exploring the Benefits of Using Annotation Tools
Utilizing annotation tools for images can yield numerous benefits for businesses and organizations:
1. Accelerating AI Training
With the rising complexity of AI models, having high-quality datasets is crucial. Annotation tools streamline the process, allowing for larger datasets to be prepared in less time, ultimately accelerating model training.
2. Reducing Costs
Outsourcing annotation tasks can be expensive. By implementing an in-house tool, organizations can reduce costs over time while maintaining control over the annotation process.
3. Improving Accuracy
Human error is common in manual annotation. Effective annotation tools for images include automated features that help mitigate errors and ensure more accurate labeling.
4. Enhancing Collaboration
As projects often involve multiple stakeholders, annotation tools that support synchronization and real-time updates enhance collaborative efforts, making it easier to align team members on project goals.
Case Studies: Successful Implementation of Annotation Tools
The impact of annotation tools for images can be better understood through real-world examples. Here are a few industries leveraging these tools effectively:
1. Healthcare Sector
In healthcare, consistent image annotation is vital for training AI tools that assist in diagnosing diseases through imaging techniques. For example, radiology departments are using annotation tools to label images for improved accuracy in detecting tumors or other abnormalities.
2. Autonomous Vehicles
Self-driving car companies depend heavily on annotated image datasets to train their algorithms. Annotation tools help in the classification of road signs, pedestrians, and obstacles, ensuring that autonomous systems operate safely.
3. E-commerce
In e-commerce, image annotation is used to tag products in images, enabling better search capabilities and improved customer experience through automated visual recommendations.
How to Choose the Right Annotation Tool
Selecting the right annotation tools for images involves careful consideration of several factors:
- Your Project Needs: Assess the specific requirements of your data annotation project, such as the type of images, the volume of work, and desired output quality.
- Budget: Evaluate the cost-effectiveness of potential tools. Some may offer a range of pricing models that can accommodate smaller budgets.
- User Feedback: Research reviews and testimonials from other users to gain insights into the tool’s performance and reliability.
- Trial Options: Many annotation tools offer free trials. Taking advantage of these can provide firsthand experience to inform your decision.
The Future of Annotation Tools for Images
The future of annotation tools for images looks promising as technology continues to evolve. With the proliferation of AI and machine learning across various sectors, the demand for accurate, efficient, and scalable annotation solutions will remain high. Innovations in automated annotation, such as image recognition and AI-assisted tagging, are expected to further streamline workflows and enhance data quality.
Embracing Automation
The introduction of automation in image annotation can drastically improve efficiency. Automation can assist with repetitive tasks, allowing human annotators to focus on more complex aspects, thereby achieving better overall results.
Enhancements in Machine Learning
As machine learning models improve, we will likely see smarter annotation tools that can learn from prior annotations and make predictive suggestions, facilitating an even smoother workflow.
Conclusion
In conclusion, annotation tools for images are not just a trend; they are a fundamental component in the process of training AI models and machine learning applications. By understanding their significance, evaluating their features, and leveraging their benefits, businesses can optimize their operations and stay ahead in a competitive landscape. Whether in healthcare, automotive, or e-commerce, embracing these tools is crucial to harnessing the power of visual data efficiently.
As the field of image annotation continues to develop, embracing cutting-edge technologies and methodologies will position organizations for success in a data-driven world.
For more information on effective data annotation tools, visit KeyLabs.ai.